Learning from Symmetry: Meta-Reinforcement Learning with
Symmetrical Behaviors and Language Instructions
Xiangtong Yao, Zhenshan Bing, Genghang Zhuang, Kejia Chen, Hongkuan Zhou, Kai Huang, and Alois Knoll
Video of simulation and real-world experiments
Real-world experiments
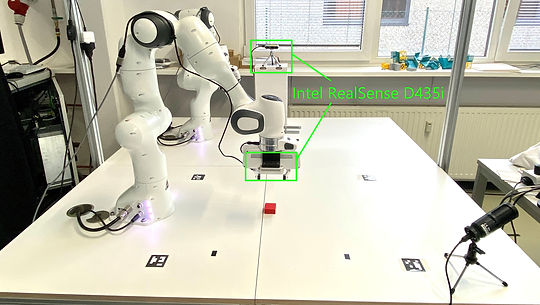
Real-world experiment scenario. We use Intel RealSense D435i cameras to locate the red object.
Push-right and Push-left
Push-right tasks
Task goal position: [0.1, 0.7, 0]
Task goal position: [0.05, 0.65, 0]
Push-left tasks
Task goal position: [-0.1, 0.7, 0]
Task goal position: [-0.05, 0.65, 0]
We adapt Panda robot to Meta-world environment. For more information, please visit
Supplementary materials
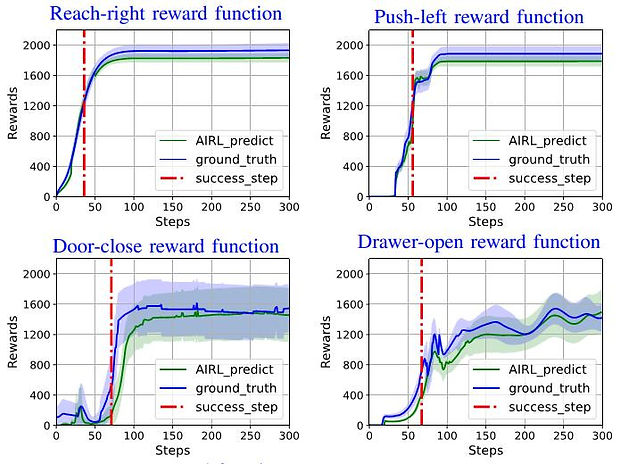
The recovered reward function of other task families
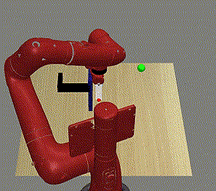
Door-close
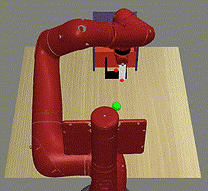
Drawer-open
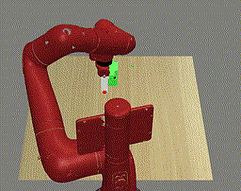
Faucet-open

Window-close
The visualisation of trained AIRL policies, each of which is trained by symmetrical trajectories generated from the Symmetric Data Generator.

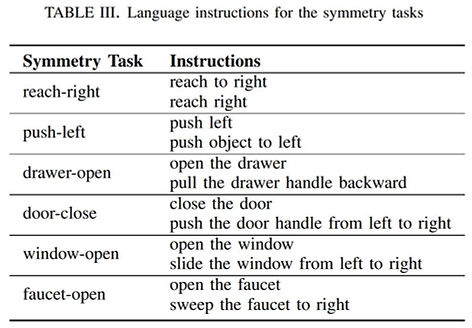
Language instructions of meta-training tasks and symmetry tasks
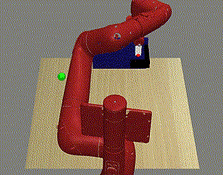
Door-open
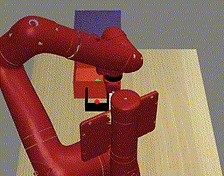
Drawer-close
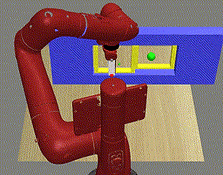
Window-open
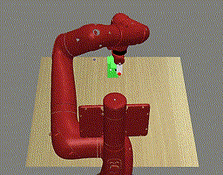
Faucet-close
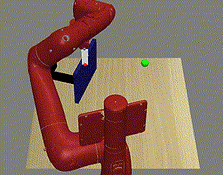
Door-close
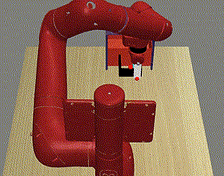
Drawer-open
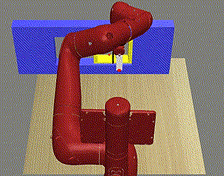
Window-close
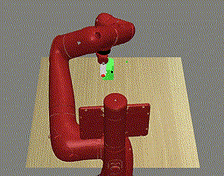
Faucet-open
The visualisation of meta-training tasks and meta-test tasks. The setting of the above tasks are the same as those of the Meta-world benchmark.